AI No Longer Just for the Big Investment Players
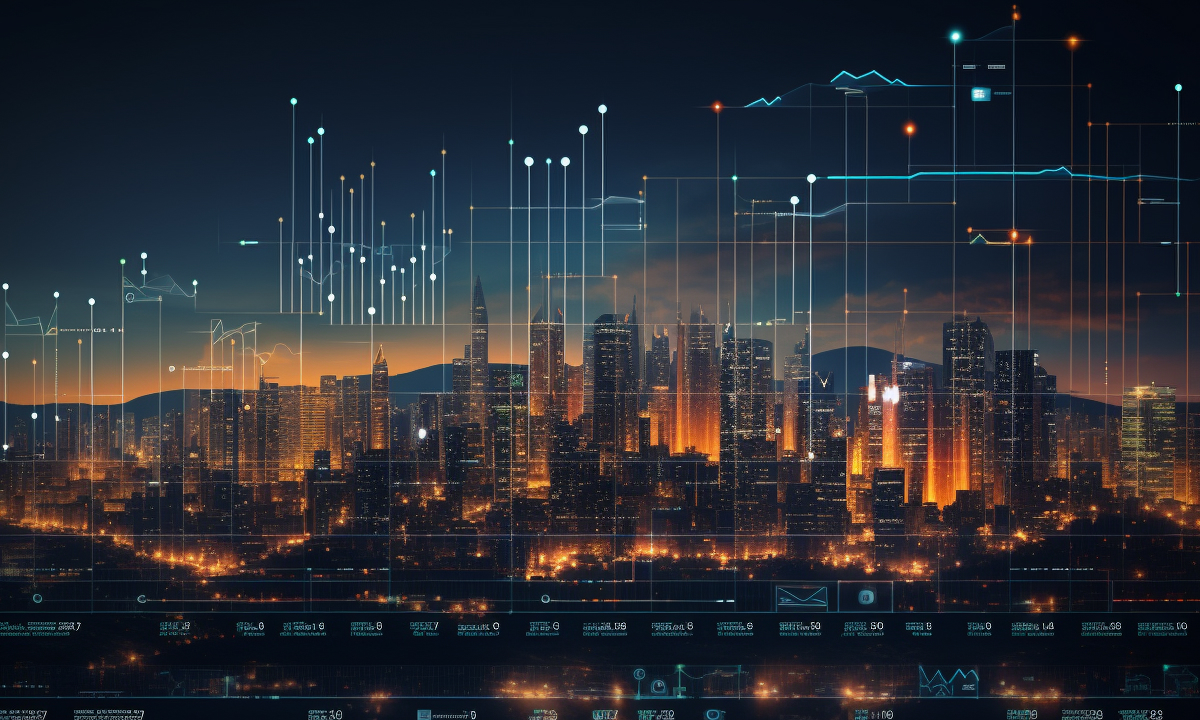
“What we're suddenly discovering with generative AI (is that) there are a lot of cognitive things that we do that are fairly routine...So you're going to go through this revolution of suddenly all those routine cognitive tasks, those will be automated." - Vanguard CEO Mortimer J. "Tim" Buckley, at a recent conference
Looking at the world of investment, some big plays are happening connected to AI and specifically, generative AI. But it’s challenging to swim through what is being said to see what is being delivered as the lines between generative AI and good ol' AI born of Machine Learning are blurred.
You've got new news from the big end of town with JPMorgan Chase & Co. (JPM) building their own language model specific to their sector called IndexGPT. Morgan Stanley is working with OpenAI to train its own model, built from years of documented intel and investment strategies. This is all built on the back of years of in ‘pre-generative’ AI investment as well, so they are simply tuning their efforts in the face of new technology.
This is great news if you're an investment firm with a market cap with 11 zeros in it – it means you're likely on it and will see value. But what if you're not that size? Does generative AI lower the entry costs to make it easier to see value?
The short answer is that it will depend heavily on how good your data is and how clear you are on the value you could get from applying generative AI, with a use case that makes sense to explore.
If you’re considering exploring AI, with generative tools, then here are three things to consider;
Generative AI can make exploring AI value easier, but you still need some specialist help.
You can get started with the proper use cases, data, and articulation of value you are likely to achieve. But you still need specialists to help ready your data and train a model or fine-tune it. Specifically, specialists that know how to ready data for ingestion and setup the environments to safely house the data and build the data model.
Natural language interrogation of data requires fewer skills, but you need to know what 'right' looks like.
Using a language model to interrogate and get new value from data is more accessible. However, if you’re looking to find insights or generate content, knowing how to tell if the model has got it right with a human expert is key. Language models in particular are great at telling you what you want to hear and accuracy can take a back seat. Ensuring there’s a knowledgeable ‘human in the loop’ when you are training your model makes all the difference.
Know what value you're going to get for the investment.
Understanding and asking the right questions to ensure you know the importance of what you're exploring is critical. Push hard to understand where the experiment will take you. Will you get faster at evaluating investments or more accurate, and therefore achieve a better operational outcome? The better you can identify the value, the stronger your chance of achieving true ROI.
Since we started providing support services for this kind of work at Deepend, it's been interesting seeing how much the not-so-big players are playing catchup, where they've invested little in AI in the past; some are starting to see value emerge that would have been considered too expensive to event attempt to attain in the past.
- Doing the work to find a valuable use case to explore, getting help to ensure you’re not only able to build the model and train it on your data, but also understand what it is showing you with the right expertise are all essential to ensuring the access generative AI is enabling truly delivers.